Podcast: Download (Duration: 1:01:09 — 49.8MB)
Subscribe: Spotify | TuneIn | RSS | More
Artificial Intelligence is already part of our lives in the tools and services we use every day. As AI development accelerates, how can authors and small businesses use it as leverage to expand income and opportunities? Ash Fontana gives some ideas in this interview on The AI-First Company.
In the intro, How GPT-3 is quietly ushering in the A.I. revolution [Digital Trends]; The Computers Are Getting Better At Writing [The New Yorker]; The Chinese equivalent on GPT-3, PanGu-α [Venture Beat]; List of AI writing tools and GPT-3 Examples.
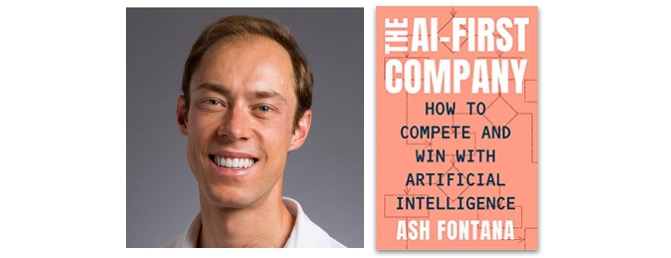
Ash Fontana is a startup investor and managing director of Zetta, an investment fund focusing on AI. He's also the author of The AI-First Company: How to Compete and Win with Artificial Intelligence.
You can listen above or on your favorite podcast app or read the notes and links below. Here are the highlights and the full transcript is below.
Show Notes
- How AI development has accelerated due to the pandemic
- How Natural Language Generation and other aspects of AI can help authors with marketing and content creation
- How AI could help stop plagiarism
- Licensing works in copyright for training AI models and the impact of AI on copyright law
- Generative Adversarial Networks
- A positive view of an AI powered future
You can find Ash Fontana at AshFontana.com and on Twitter @ashfontana. You can also find the book website at TheAIFirstCompany.com
Transcript of Interview with Ash Fontana
Joanna Penn: Ash Fontana is a startup investor and managing director of Zetta, an investment fund focusing on AI. He's also the author of The AI-First Company: How to Compete and Win with Artificial Intelligence. Welcome, Ash.
Ash Fontana: Hey, Joanna. Thank you very much for having me.
Joanna Penn: I'm excited to talk to you today. Let's start with a quote from the book. ‘We thought AI was just around the corner for a long time, but it turns out that it was just getting started.' Now, many people in my community think, ‘AI will happen in a decade or longer,' but,
What are you excited about right now and have things accelerated because of the pandemic?
Ash Fontana: In many ways, AI will happen in a decade or longer and it'll keep happening for many decades going forward. We're just at the start of what I call the AI-first century. We're only partway through that.
So bringing it back to today, where are we, and this AI-first century, and what's exciting today, and what's changing today, I'm still excited by a lot of the simple stuff, what we call supervised and semi-supervised systems in the field of AI.
That is a human is manually training the system, telling it what to do next, giving it examples to learn from, etc. And these systems are just so good at picking out things that you tell them to pick out like clauses in a contract or anomalies in a stream of clicks on your website.
And there's so much opportunity to just apply all of these things, these systems to all sorts of things today. I guess another relatively simple, at this point, or at least over the last five years, it's become relatively tractable for most people, way to apply AI is providing a view on something using perception technologies.
So to bring it to a real-world example, figuring out what's on a shelf in a grocery store rather than having someone have to walk the aisles at 4:00 a.m. with a little machine. Or if someone is standing in front of a forklift on a factory floor, you want to know that so that you don't hit them.
There are just endless use cases in the real world from manufacturing to mining using the technology we have right now.
I think if you were to consider what's next maybe not the next couple of years, but very soon after, the real opportunity is modeling complex systems. The way you do that is you construct a relatively simple, what we call an agent-based system.
You say you have an agent, a little character in a story, so to speak, you give them incentives and having a little environment with their constraints and you can start doing things like saying, ‘How will this economy, or this biological system, or this virus behave if we give it these incentives? We have like some idea of the mechanism, but not totally, let's see it play out.'
I think there's potential to apply AI to understand some of these complex systems that we really don't understand today. So that probably they're two parts of the answer. I'm excited by some simple stuff today and excited next up by modeling complex systems.
You asked about the acceleration of the pandemic, and I think things have definitely accelerated. Essentially, we've had to figure out how to do some tasks like warehousing without people coming into contact with each other or without people at all.
What we've had to do is use AI to map those processes in certain ways and then let AI learn those processes so we can use it both to map, so observe something and figure out, okay, what is the usual pattern of operations here in this factory, and then learn, okay, what is the output we're trying to achieve, and then even carry out those processes and maybe learning things about that process that we missed.
So, again, thinking about running a factory floor and all the things that happen, can't really do that in the pandemic. People are home and people are not really able to come into contact with each other at work. So we've seen a huge acceleration in the adoption of technologies that let you do that.
We've also, quite obviously, had to come up with solutions to some really big problems like designing new drugs. I won't go into that now, but AI has definitely played a big role there.
Looking forward and just to wrap this up, on the consumer side, there's some really exciting ways people are using AI to help people learn things faster and quicker. So learn a new skill, learn, whatever it is, like how to solve their mathematics problems in their grade seven homework all the way through to how to re-skill for a new career as people figure out what they need to do to re-enter the economy. So I'm pretty excited by that too.
Joanna Penn: I think this is the exciting thing, like you talked about, they're modeling complex systems and some of the biggest problems of our planet, healthcare, environment, education, these are the things that could be transformed in such a positive way.
Of course, this is a podcast for authors and writers. Actually, most people don't care about writing books. This is not a big application of AI, but given that's my audience, and you're an author, obviously, you have a new book and the audience is authors. And as you said enhancing humans is something that it does right now.
How do you see natural language processing and generation as part of ‘The AI-First Company' because that is accelerating a lot?
Ash Fontana: I think I'll answer this question about how natural language generation can be used in companies in general. And then maybe later on, we can jump into authors and creative entrepreneurs, in particular.
Natural Language Generation, as you've covered on this podcast before and as people have read in the news over the last year or two, is really picking up in terms of its ability to generate large segments of text rather than just a little sentence at a time, which we've been able to do for a while.
Generating sensible sentences in long sequences has been very, very hard to do. But with both developments on the modeling side and on the computing side, more resources available, etc., we've made a lot of progress there, which is really cool.
And you can think of how this could be useful in lots of different ways in most companies, regardless of the industry, getting started on a marketing piece an AI could write, or a natural language generation system could write 80% of the piece, the first draft, and then you craft it from there, generating an agenda to make meetings more productive.
We can look at who's attending the meeting, look at what they've written in their documents from previous meetings and generate an agenda or a starting document for our next meeting. They can map out charts, organizational charts. They can get most of the way in responding to people.
So I think in most companies, natural language generation systems can just keep up the flow of communication without totally taking it over. And there's a lot of nuance into how it would do that. In industries like media and marketing can certainly utilize natural language generation more heavily.
It's already doing so, generating articles in fields where there's a lot of structured data, so sports and finance, which also happened to be fields where delivering articles close to real-time is really important or more used, increases the utility of the article. So that's quite cool to see.
[From Joanna: You can find all my articles and podcast episodes on AI and writing here.]
I think in terms of the publishing industry, in particular, it's remarkable that a lot of people in the industry don't really have a great idea of what sells at a very specific level. Do books with orange covers or blue covers sell better? That's a question you could ask most people in the publishing industry and they won't really give you an answer to it, they don't really know.
But it's a question you could ask an AI very easily and it could very easily answer. Or even just looking at the content. Do books with checklists do better than books without checklists? Do books with long chapters or short chapters, lots of smaller chapters, fewer bigger ones, by genre?
There's all sorts of information that the publishing industry could generate using natural language processing and then also natural language generation. But we can go more and more into that, and you and I probably have lots of ideas there, but I think that's probably where I'd start.
Joanna Penn: One of the problems is that people who have an interest in AI or people who have any sort of technical background don't go into publishing and then people like yourself and other more technical people might write books and then talk to people in publishing, but the people working in publishing are not usually technical people and they also think that's kind of blasphemous to even consider the idea of split testing a different color.
You did tease us there, about talking about specifically for authors and writers. Let's just get into that now.
Ash Fontana: I'll start with where you finished there, which is the idea of split testing or whatnot. The most exciting use of AI in the industry, not just natural language processing and generation, including natural language generation, but the most exciting use of AI is the most boring but most impactful, which is marketing your book.
Things like writing short tweets, and blog posts, and figuring out which segments of the book are good to turn into a shorter piece that can be distributed for free as a teaser for the book.
Generating marketing content rather than core content, rather than writing your book for you, I think that is the biggest opportunity for authors to utilize AI.
And, again, it's not as cool as thinking about an AI that can write a book, but I just don't think AIs are going to write good books for a very, very long time. I do think a lot of authors need a lot of help in marketing, and especially if you're going down the path of self-publishing or whatnot, you'll need a lot of help. There's a lot of opportunity in a lot of the challenges there, and that's where AI can jump in.
Even if you think about the way people consume content and the way that media is changing, people want content in different sizes and on different media. So people want a one-tweet summary of a book. they want a blog press summary. They want a five-page summary. They want, you know, a chapter-level summary.
AIs can generate all of those for you, as you probably know as an author going through and generating all of those summaries, distilling everything you've written down to that level is really hard, but AI could help with that. And then you can distribute it across all sorts of different media.
You could have an AI read it out for you in a five-minute podcast that someone can listen to while they're doing the dishes, or you can have it read out your entire book or even a slightly shorter version of your book that's a one-hour version. Or it can generate an audio excerpt with a bit of a video, even generate its own illustrations to put in that video that you could post on Instagram.
If you think about both the summarization and the language generation side of things, and then if you think about the way people are consuming content today, and then the imperative for an author to sell that content, to sell their book, there's lots of really cool stuff that you could do, combining a lot of technologies and distributing the output of those AI as across lots of different media types to basically sell more books, I think.
That's really what you need to do as an author so you can keep supporting yourself and bringing new ideas into the world and pushing humanity forward.
Joanna Penn: I think people will be encouraged by you saying it won't write good books for a long time because that's what people are worried about. But what is happening, of course, is people are using tools like GPT3 to help them write faster.
And, of course, we've got AI translation. I'm just about to do my fourth German book with AI translation first draft. So we are looking at expanding the amount of work created with these AI tools.
Going into data, because the book has a lot about data, which is fantastic because this is an engine of the AI age, owning it, generating it, using it to power models. And you mentioned data brokers and licensing data, which I'm very excited about.
As you mentioned, authors and publishers have a lot of data, a lot of words, and intellectual property. What do you think are the prospects for licensing books in copyright for training language models? Has the horse bolted already, as in people are just doing this with no permission as such? Will licensing be ready for that kind of thing?
What do you think are the prospects for licensing books in copyright for training language models?
Ash Fontana: This is such a good question because it involves so much opportunity, but so many challenges in that if you think about the businesses that have been built so far, licensing and brokered data, structured data, they're huge.
Bloomberg and Equifax and all these other companies make so much money by packaging up and selling structured data, data on people, on financial markets, on whatever else. But very few people have figured out how to package up and sell unstructured data like text data in quite the same way.
Some of the technologies that we've talked about here today may allow you to do that. But as you said, the challenge is how do we do that in a way while still protecting the core asset?
And you're right, today most people train these big language models on the tomes of publicly available text out there, whether it's out of copyright text or whether it's text that's in the public domain, written by a government agency or just published for free online. That is the text people use to train these language models today.
But, of course, if you're trying to train a model to write a really good piece of modern fiction where you want to train that on a piece of non-fiction, that's still on the copyright.
There is an opportunity here to, I think, build businesses, whether it's an individual author selling their content or whether it's a business selling multiple authors' content around selling unstructured text data to train the models or even selling the models on top of them themselves.
We have to be really diligent as writers to make sure our books aren't machine-readable until we figure out how we can sell that content because otherwise crawlers will crawl them and use them effectively to train a model and then sell that model or sell the output of that model.
That's pretty hard to do, but we have to continue to innovate on both sides of this, which is both on the natural language, understanding and generation side, but also on the digital rights management side. That's going to be hard, but we have to not forget to do that.
Plagiarism is rife and it's been rife forever. And so on the one hand you could say, ‘Oh, well, there are no new ideas anyway, so we shouldn't take it very seriously.' But on the other hand, people's ideas are genuinely stolen all the time. I've had it happen to me, I'm sure lots of people out there listening have had happen to them.
There's a huge opportunity to use AI to finally end plagiarism.
We can look at results from a competition that Kaggle ran to grade essays many years ago. Kaggle, for those who don't know, is the world's biggest community of data scientists and machine learning engineers, and they compete to solve really hard problems, problems of identifying dark matter in space or figuring out if someone's got a heart condition.
They use machine learning to do that. And one of their competitions on that was grading essays and there'd been various other approaches out there that figure out if things are being plagiarized and grading them accordingly. I think there is hope in using AI to make sure people's ideas on stolen because AI can analyze huge volumes of text and match them up pretty easily.
Joanna Penn: I think about this a lot. What's interesting about GPT3, for example, is it's something like 98% is not plagiarized. Even though it's trained on all this material, it comes up, ‘original work.'
And so I agree with you. I think there needs to be some kind of almost watermark. They're talking about it with deep fakes, a way that is put on the files in some form so that you can tell what is generated and what is real, but this is becoming harder and harder, obviously.
The other thing I did want to ask about, some of the more legal side because, of course, copyright law and protection of intellectual property assets.
China has granted copyright to an AI owned by Tencent, I believe, but this hasn't happened in the U.S, U.K., Australia, etc. It seems to me that already this is going to become an issue because the legal aspects move so slowly compared to the technology.
What are your thoughts on copyright law and protection of IP?
Ash Fontana: The starting point is that anything generated by an AI is definitionally derivative. It is data that's gone into a model, that's done some sort of, calculus…usually, not always, and derived something. We have concepts of derivative works and whatnot in copyright law today.
I think there's a lot of different ways that courts maybe have and will apply those concepts that we already have to the output of AIs, to the output of these machine learning or otherwise models.
Now, that's just a starting point. It does get a little bit complicated and nuanced, I guess, in that the output of, on the one hand, heavily-designed, almost rules-based system, which you could call an AI, in some cases could be said to be the work of the designer of that system, the data scientist or machine learning engineer. And so you could say that, well, that's quite clearly the work of that person, and anything generated by the AI is owned by that person or that entity that designed that system.
The good thing is, in those cases, those systems are quite explainable and therefore the work quite truly the work of the designer of the system, they write the rules and the system generates things, predictions, whatnot, based on those rules. So that's what we've got on the one hand.
On the other hand, we've got systems that not just learn the underlying structure and whatnot, but learn the rules themselves. And so there's just many, many layers to the system.
Maybe it's a deep learning system, which is what deep learning means, like many, many layers to the network that's learning things across pieces of data. And it's less straightforward there, like how you would attribute the work.
Is it attributed to the design of the system? Is the data on which it's trained at that point really playing much of a role in generating the output of that system? Probably not. And so this is where it becomes a really difficult question of degree of authorship.
Certainly, the input data was required, and certainly, some design was required in some of those cases, but certainly you can't track back to those two things, the data and the design of the system to explain the output of that system. And so, therefore, could you say the owner of the data or the designer owns any of the output of the system or should own the output of a system. That's going to be really hard.
I guess in summary, a lot of the simpler AIs, I think, will be protectable through traditional concepts or existing concepts of copyright law but some of the newer ones, it's going to be pretty hard.
Joanna Penn: I agree. I was quite militant on this last year, but my attitude has changed as I've learned more and I feel now that you won't be able to tell, so I might want to license my data.
I've got over a million words of fiction, but a million words of fiction in book form, that's not much when you think about how much things take to train models. So if my work is sucked into a thing or your book is sucked into a thing, it will disappear in the mass of other models and we'll never even know that it was used.
So I've kind of been like, ‘Okay, well, in that case, let's focus on a different angle, which is embracing this stuff and really looking to how we can use it,' which is now my attitude is, ‘Let's think positively and get on with using this stuff instead of trying to prevent it being used.'
I do want to ask about the more creative ideas. I find the generative adversarial network, even though the word adversarial is difficult, I think, for creatives, but the GAN idea, fascinating, as it's similar to this idea in my head of the creative first draft, and then the editor, the sort of, ‘I'm going to create this and then I'm going to edit it. I'm going to create this and edit it.'
How do you think this type of Generative Adversarial Network (GAN) idea might help creative entrepreneurs?
Ash Fontana: Oh, in so many ways. Firstly, it just conceptually. It's so nice. I think of it like a debate. You've got a for and against, you've got two people debating and that's how I would argue most human-based politics and governance and development has happened by people collaborating and people improving each other's ideas.
It's such a powerful concept. I think the first way it can help creative entrepreneurs and companies is in generating things that are just hard to otherwise get. So, let's just say you need illustrations of 10 different types of chairs for your book about chair design or some industrial design book. Maybe that's an easy thing to get, and so therefore maybe it's not a great example.
But if you say to a GAN, ‘All chairs have four legs and a seat and a back, now go and just generate a whole bunch of them,' it can do that. And a more pointed example would be something like generating lots of pictures of different vehicles or whatnot that may be very futuristic and may still resemble a vehicle, but being nothing like we've ever thought about before if you want to write a book about the history, or the future, the history and future of vehicles, or of warfare, or something like that.
So that's on the image side. And, of course, you can think about this on the tech side, just generating ideas that are based on concepts that we already have, but the output of which is in the models output of which we've never really thought about, which is very surprising.
You can think of human-machine interfaces where you're going back and forth with one of these GAN-based systems and coming up with new ideas, whether they're new ways to look at an equation, new angles to look at an image, different light, different characteristics, or different ways to throw words together in a paragraph.
I think it's really powerful. It's a really powerful concept. And they're inherently creative, is what I'll say again.
Joanna Penn: On those images, is that similar to OpenAI's DALL-E that they've just introduced? You mentioned the chairs there, it said, ‘Create a chair, an avocado chair,' and it generated a whole load of images of an avocado chair, some of which were obviously unusable, but some of which were truly original, which is just fascinating.
Ash Fontana: Yeah, it is. It's really fascinating. It's a combination of methods, I think. I haven't read that paper, but certainly seen it. It's a combination of methods, but that's an example.
Let's take something that we already have a conception of and let's generate a version of it, or two things we have conceptions of and generate something that we've never seen before and let's think about what that means.
Obviously, an avocado chair doesn't mean anything, but you can think of something like, ‘Well, let's take this concept and that concept, here's a paragraph on age. What do you think those concepts would mean if we talk about them together?' And that's the sort of thing a GAN-based system could generate for you.
Joanna Penn: As I've been trying out the beta of GPT3, it's fascinating that it comes up with stuff that I would not have thought of and using that to enhance my thinking as opposed to just copying it and pasting it, I'm using it more to enhance my thinking.
And that's what you were talking about at the beginning, that sort of enhancing human work. But I mean, we're talking about lots of exciting things and what I really noted in the book was…obviously, your book is really aimed at bigger companies, so ‘The AI-First Company', but, of course, we're all micro companies.
To people listening, I'm a micro company. It's just me. But I want to use all these tools. Now, you've mentioned lots of ideas.
Are there tools available right now for non-technical people who want to make more revenue but don't want to have to employ a programmer or a data scientist?
Ash Fontana: Yeah. Very much so.
The book, I think, and I hope, is very relevant to people with fewer resources. One of the premises of the book is you don't need a Ph.D. in machine learning to get started with AI and you don't need the multimillion-dollar budgets of companies like Google, and Facebook, and Amazon that they have to devote to specific projects to get started with AI.
You can get started right now because you've probably got a dataset, whether it's your million words of fiction or whether it's all the clicks and interactions on your website or whatnot. That is perhaps able to generate or from which you're able to generate some predictions about something that could be valuable to someone. And so, yeah, people can absolutely get started with AI with what they have today.
There are lots and lots of tools available to non-technical people. It's amazing to either use some of the tools provided by vendors today to turn lots of speech into text or to translate all of your text in one language into another language, which you touched on before.
I will say it's such a huge opportunity, both commercially, but also for the good of the world to have all of the non-English speakers in the world to be able to access all the English-written content would be amazing. There's so many people out there that could learn so much if they were able to access that content.
There are lots of off-the-shelf tools provided by vendors, but it's actually pretty straightforward to build a lot of your own stuff using tools provided by companies like Google and Amazon. I haven't been paid to write computer programs in a long time, arguably ever. And I can go on Amazon's site and start playing around with a lot of their products and APIs and some of their coding gyms, so to speak, to train AI's. And it's very straightforward.
There's a lot of really good stuff out there. And then, of course, the computing power and everything else is more freely available now.
The opportunity for one-person companies to be AI-first is there just as it is for million and thousand-person companies.
Joanna Penn: And certainly, I really enjoyed the book. I found it really useful. I also think there's almost a language aspect of this. I feel it's almost like the finance industry; if you don't understand some of these terms, then you think it's more complicated than it is.
Whereas, actually, for example, you mentioned that the Amazon tool, so that's AWS, people can go to Amazon AWS, and you go on their AWS homepage now and it is full of things you didn't even know you could do easily. And as you say, they have made it aimed far less at technical people now. There are lots of ways that you can do so.
The other thing I've been thinking is perhaps this is all just architecture. And as you say, we're in the middle of this AI century, will even use the phrase AI as the years go by or will it be like the internet? You didn't publish your book on the internet, you published it on Amazon or your publisher put it on Amazon. I don't say I put my books on the internet.
Will AI become part of architecture so we won't talk about it in the same way as the companies move forward?
Ash Fontana: Yeah, I think so. Absolutely. I think that it is something that will undergird all of the technologies that we use, all of the software that we use more pointedly will become just that little bit more predictive.
You won't just open your video conferencing application and see someone else's picture in front of you directly from the camera stream. It will have been processed by all sorts of smart systems to provide you with a better quality picture, better quality information about maybe what they're feeling or what they're emoting at that point. You might get an automated transcript.
All of those things sound like things that might be in the future but they're all actually happening right now.
And that's just your video conferencing software. Your software that you use to manage your blog might write your blog for you, and then might write it in 10 languages for you, and then might figure out the best place to pay for a link back to that blog or whatever else.
Your accounting software won't just take the entry you put into it and spit out a report. It'll just ingest all of the information it can from your bank account and email and whatever else and do it all for you. It'll just become invisible. It'll become completely invisible.
That's what we hope that a lot of these technologies are abstracted away so we can live our lives just with more time and money.
Joanna Penn: Yes, hopefully. I think that is the question I want to end with really, which is the ideal is, as you say, we'll have more time and more money and life will be amazing. And we're obviously techno-optimists in this way.
But a lot of people are worried. For example, you talked about the warehouse back in the beginning and what we have seen is machines don't get sick. So if you replace humans with the machines, then humans don't get sick, but humans also don't have a job. I feel like that is the fear that people have.
In the creative community, there's a fear of a tsunami of AI-generated content that pushes out the real authors as such.
How come you're so positive about this? And how can others adopt this more positive-looking mindset?
Ash Fontana: I always come back to two things, leverage and optionality, which is all technology is, it's a lever for you to do more with less. It's in a very Archimedean sense, whether it is one of the very early pieces of technologies such as a wheel, or a scale, or an ax, or a trap.
All of those things actually give us physical leverage over our environment so we can carry more with the same muscles on our body or so that we can reap more with the same amount of energy expended. That gave us leverage over our physical environment.
Now we've moved to having leverage over intellectual things and that allows us to develop more intellectual products for our own consumption for enjoyment or for the purposes of making a wage.
That's all technology is; it's a form of leverage that gives you more from less.
AI is just like any other of those pieces of technology. It's a way to give you predictive leverage. So it gives you leverage, not over your physical environment and not over your intellectual environment, it gives you leverage over time because it can learn from the past to predict the future.
It can help you bring things forward, whether that is, ‘Should I spend this money now or not, or should I get this insurance policy or not? Or will I have food on the shelf in a month, or should I order it now?' AI can help you develop more certainty around the future so that you get leverage over time.
And that's really, really cool. It's a bit abstract, but you can let that sit for a bit and think about how that might improve everyone's lives. And so that's one thing I always think about with technology, whether it's AI or software or networking technology like the internet or otherwise.
The other thing I always think about is optionality, like, look, take it or leave it. It's totally fine to not have AI in your life.
I actually don't adopt AI for a lot of things in my personal life.
I don't use Spotify that suggests music to me because I really enjoy the process of discovering music and discovering artists. I don't use AI to figure out what movies I want to watch. I've never used Netflix in my life because I enjoy the process of talking to my friends who study film and whatnot to figure out what movies I want to watch.
It's totally fine to pick and choose where you will adopt it. And we certainly should be aware of that. I don't use my phone from a whole day a week and for a certain number of hours per day.
We have a lot of technology in our lives. Just pick up the right tool at the right moment when you want to achieve whatever goal you have and when that's not the right time or moment, then just leave it on the shelf. That's how I think about these things.
Joanna Penn: Fantastic. The book is The AI-First Company.
Where can people find you and the book online?
Ash Fontana: Sure. The book, you can find, obviously on Amazon and all good booksellers. The website is ai-first.org. You can also find the book website at TheAIFirstCompany.com
And I am Ash Fontana on Twitter and LinkedIn and all of those other places on the internet. So fairly straightforward. I hope people enjoy it and I hope lots of different types of people enjoy it, whether they're working by themselves, or working in a really big company, or working in their family business.
Hopefully, it's practical enough that you can take some of the checklists, and illustrations, and frameworks, and apply them to something you're working on right now, or thinking about, or what you want to do next. That's why I wrote it, to help everyone, you know, every day. So hopefully it does that.
Joanna Penn: Fantastic. Well, thanks so much for your time, Ash. That was great.
Ash Fontana: Thank you.
Leave a Reply